Private AI, We Salute You!
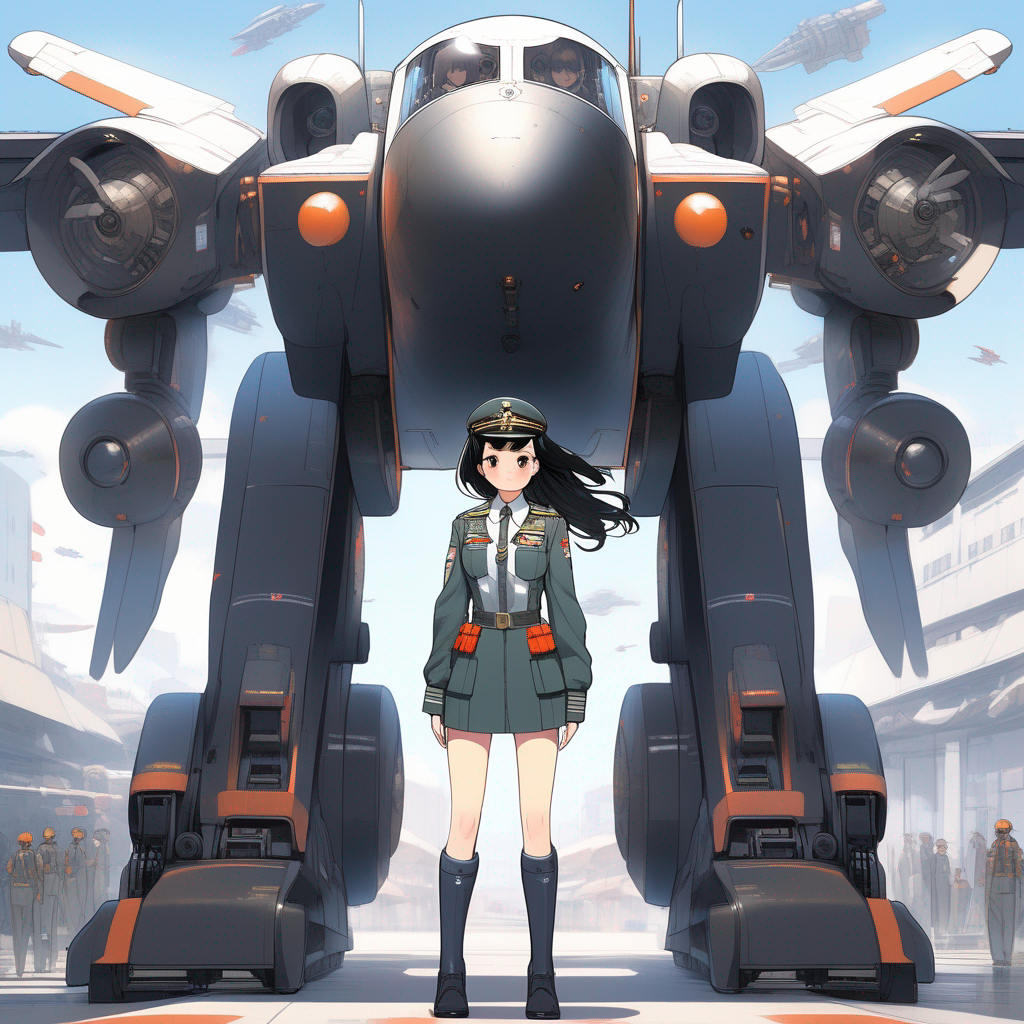
So VMware made the case this morning that data privacy is the big obstacle in the way of enterprises leveraging new generative AI solutions (e.g., ChatGPT). If “private AI” is so important especially with generative AI (genAI) large language models (LLM), why is VMware at the same time touting their new genAI solutions for AIops that must be trained with non-private aggregated data?
Without having had any time to really dive in, there seems a big and obvious disconnect between VMware stating why private AI is so important, announcing their joint work this morning with NVIDIA on outlining Private AI architecture, while at the same time announcing their not-so-private generative AI solutions for AIOps in VMware Tanzu, NSX and Workspace One. I mean not-so-private in a gentle way, but so far beneficial AIOps solutions (from any vendor) have necessarily been trained on a larger corpus of collected aggregated customer data – not strictly private data. That’s actually why AIOps has value – it’s learned from every user and each user can benefit from the total deployed experience.
Now a vendor can say that they’ve trained their non-LLM Machine Learning (ML) analytics only on extracted meta data, normalized and anonymized (and scattered with onions? Sorry, missed lunch watching the keynote and thinking of waffles). That extraction process is generally acceptable and won’t really have much of chance of leaking or sharing anyone’s IP. But here now with gen AI-style AIOps there really is a key difference. Generative AI is based on large language models (LLM)’s with billions of nodes inside them trained on huge volumes of natural language input documents. If you have ever played with an LLM (e.g., ChatGPT), you quickly appreciate that they can be eerily comprehensive, very well spoken, but also mysterious and flaky as hell. You can add guardrails, prune the domain, layer on post-processing filters, but I’ve yet to see an LLM that can’t be tricked into naughty conversations through clever prompt engineering (and let’s not even talk about the dangers of potentially hallucinating AIOps offering one-click remediations for enterprise-wide IT issues).
I’m sure VMware’s genAI models have been thoroughly guardrailed and tested. VMware wouldn’t release stuff it didn’t believe was solid. But I also feel safe in predicting genAI used for key enterprise AIops will fail someone spectacularly somewhere soon.
As to the first concept above, more commoditized Private AI for everyone, hey we salute you! Looking sharp in that new VMware/NVIDIA uniform! Only thing is, see, privates are at the very bottom of the hierarchy and have to salute everyone else first. At least its enlisted in the game. Any market messaging SNAFU aside I assume this project will lead to validatable reference architectures from the various well-known partners involved like HPE and Lenovo. That will prove very interesting because we can then directly compare and compete those solutions. And I’m sure we will soon have more to say about the overall efficacy and efficiency of building AI infrastructure on virtual/shared resources versus more HPC-like optimized clusters. I mean, sharing expensive GPU’s is great and can help you maximize their total utilization, but GPUs are still mostly bottlenecks in serious AI workload processing (note: not the only bottleneck, HPC-performant storage is key here too). If you are depending on AI, you’d only really want to share bottlenecking resources through scheduling, not with dynamic virtualization competition.
(Disclaimer: We didn’t get an analyst insider pre-brief from VMware on this AI news, so this is an early first-take based on today’s Explore keynotes)