Big Data Defined by the V’s
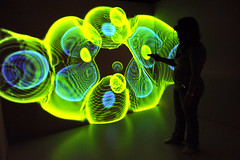
There are lots of definitions of Big Data. Most of them are fuzzy marketing speak along the lines of “Big Data is just bigger than your old data, too big to deal with the same way you dealt with data before.” Amusingly a lot of examples being given for “historical” Big Data successes are based on traditional data methods and technologies applied overlarge amounts of traditional data.
Clearly there is something new happening with the way we can get value out of very large data sets, but it’s really hard to see what the line really is between Big Data and not-so-Big Data. Ironically most pundits seem to be saying we can spot Big Data the same way we know what’s obscene – we’d simply recognize it when we see it. The irony of course is that Big Data is just too big to see, or visualize as it is.
Think how big a picture it would take to show a 5 Pb Big Data set at one pixel per data point.
Big Data by the V-words
I’ve read more than a few definitions that talk about some clever V-word characteristics that Big Data scientists need to be concerned with:
- Volume – Obviously Big Data is Big.
- Variety – Many identified Big Data sets are internally heterogeneous (e.g. big data documents). The data isn’t collected or authored according to a single master schema.
- Velocity – Big Data sets tend to grow rapidly, even as we use them. Implies some dynamic and possible real-time behavior as well.
I’d add a fourth V:
- Veracity – Or rather, the lack thereof. Raw Big Data is often not verifiable/verified nor validated (until processed for that goal specifically, e.g. security fraud). Analysis can’t always be duplicated (as data keeps growing/changing). Duplication, omission, and general incompleteness are to be expected.
It may be impossible to repeat the same analysis definitively on a truly big “big data” set. If results can’t be exactly reproduced (or explained back to raw data), they can’t serve as literal truth.