Smarter storage starts with analytics
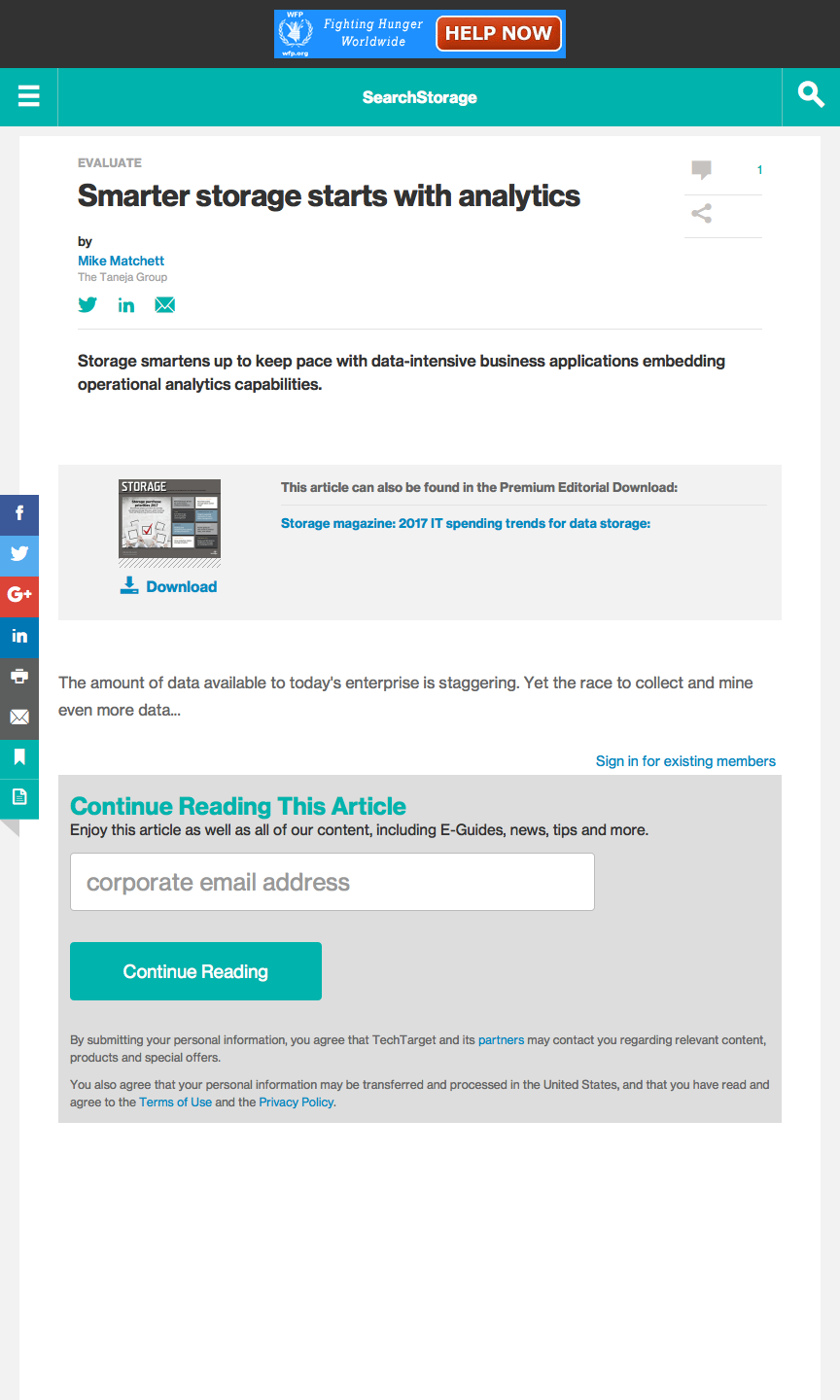
An IT industry analyst article published by SearchStorage.
Storage smartens up to keep pace with data-intensive business applications embedding operational analytics capabilities.
Mike Matchett
The amount of data available to today’s enterprise is staggering. Yet the race to collect and mine even more data to gain competitive insight, deeply optimize business processes and better inform strategic decision-making is accelerating. Fueled by these new data-intensive capabilities, traditional enterprise business applications primarily focused on operational transactions are now quickly converging with advanced big data analytics to help organizations grow increasingly (albeit artificially) intelligent.
To help IT keep pace with data-intensive business applications that are now embedding operational analytics, data center infrastructure is also evolving rapidly. In-memory computing, massive server-side flash, software-defined resources and scale-out platforms are a few of the recent growth areas reshaping today’s data centers. In particular, we are seeing storage infrastructure, long considered the slow-changing anchor of the data center, transforming faster than ever. You might say that we’re seeing smarter storage.
Modern storage products take full advantage of newer silicon technologies, growing smarter with new inherent analytics, embedding hybrid cloud tiering and (often) converging with or hosting core data processing directly. Perhaps the biggest recent change in storage isn’t with hardware or algorithms at all, but with how storage can now best be managed.
For a long time, IT shops had no option but to manage storage by deploying and learning a unique storage management tool for each type of vendor product in use. This wastes significant time implementing, integrating and supporting one-off instances of complex vendor-specific management tools. But as managing data about business data (usage, performance, security and so on, see “Benefits of analytical supercharging”) grows, simply managing a metrics database now becomes a huge challenge as well. Also, with trends like the internet of things proliferating the baking of streaming sensors into everything, key systems metadata is itself becoming much more prolific and real-time.
It can take a significant data science investment to harvest the desired value out of it.
Perhaps the biggest recent change in storage isn’t with hardware or algorithms at all, but with how storage can now best be managed.
Storage analytics ‘call home’
So while I’m all for DIY when it comes to unique integration of analytics with business processes and leveraging APIs to create custom widgets or reports, I’ve seen too many enterprises develop their own custom in-house storage management tools, only for those eventually becoming as expensive and onerous to support and keep current as if they had just licensed one of those old-school “Big 4” enterprise management platforms (i.e., BMC, CA, Hewlett Packard Enterprise [HPE] and IBM). In these days of cloud-hosted software as a service (SaaS) business applications, it makes sense that such onerous IT management tasks should be subscribed out to and provided by a remote expert service provider.
Remote storage management on a big scale really started with the augmented vendor support “call home” capability pioneered by NetApp years ago. Log and event files from on-premises arrays are bundled up and sent daily back to the vendor’s big data database “in the cloud.” Experts then analyze incoming data from all participating customers with big data analysis tools (e.g., Cassandra, HBase and Spark) to learn from their whole pool of end-user deployments.
Benefits of analytical supercharging
Smarter infrastructure with embedded analytical intelligence can help IT do many things better, and in some cases even continue to improve with automated machine learning. Some IT processes already benefitting from analytical supercharging include the following:
- Troubleshooting. Advanced analytics can provide predictive alerting to warn of potential danger in time to avoid it, conduct root cause analyses when something does go wrong to identify the real problem that needs to be addressed and make intelligent recommendations for remediation.
- Resource optimization. By learning what workloads require for good service and how resources are used over time, analytics can help tune and manage resource allocations to both ensure application performance and optimize infrastructure utilization.
- Operations automation. Smarter storage systems can learn (in a number of ways) how to best automate key processes and workflows, and then optimally manage operational tasks at large scale — effectively taking over many of today’s manual DevOps functions.
- Brokerage. Cost control and optimization will become increasingly important and complex as truly agile hybrid computing goes mainstream. Intelligent algorithms will be able to make the best cross-cloud brokering and dynamic deployment decisions.
- Security. Analytical approaches to securing enterprise networks and data are key to processing the massive scale and nonstop stream of global event and log data required today to find and stop malicious intrusion, denial of service and theft of corporate assets.
That way, the array vendor can deliver valuable proactive advice and recommendations based on data any one organization simply couldn’t generate on its own. With this SaaS model, IT doesn’t have to manage their own historical database, operate a big data analysis platform or find the data science resources to analyze it. And the provider can gain insight into general end-user behavior, study actual feature usage and identify sales and marketing opportunities.
Although it seems every storage vendor today offers call home support, you can differentiate between them. Some look at customer usage data at finer-grained intervals, even approaching real-time stream-based monitoring. Some work hard on improving visualization and reporting. And others intelligently mine collected data to train machine learning models and feedback smarter operational advice to users…(read the complete as-published article there)