IT pros get a handle on machine learning and big data
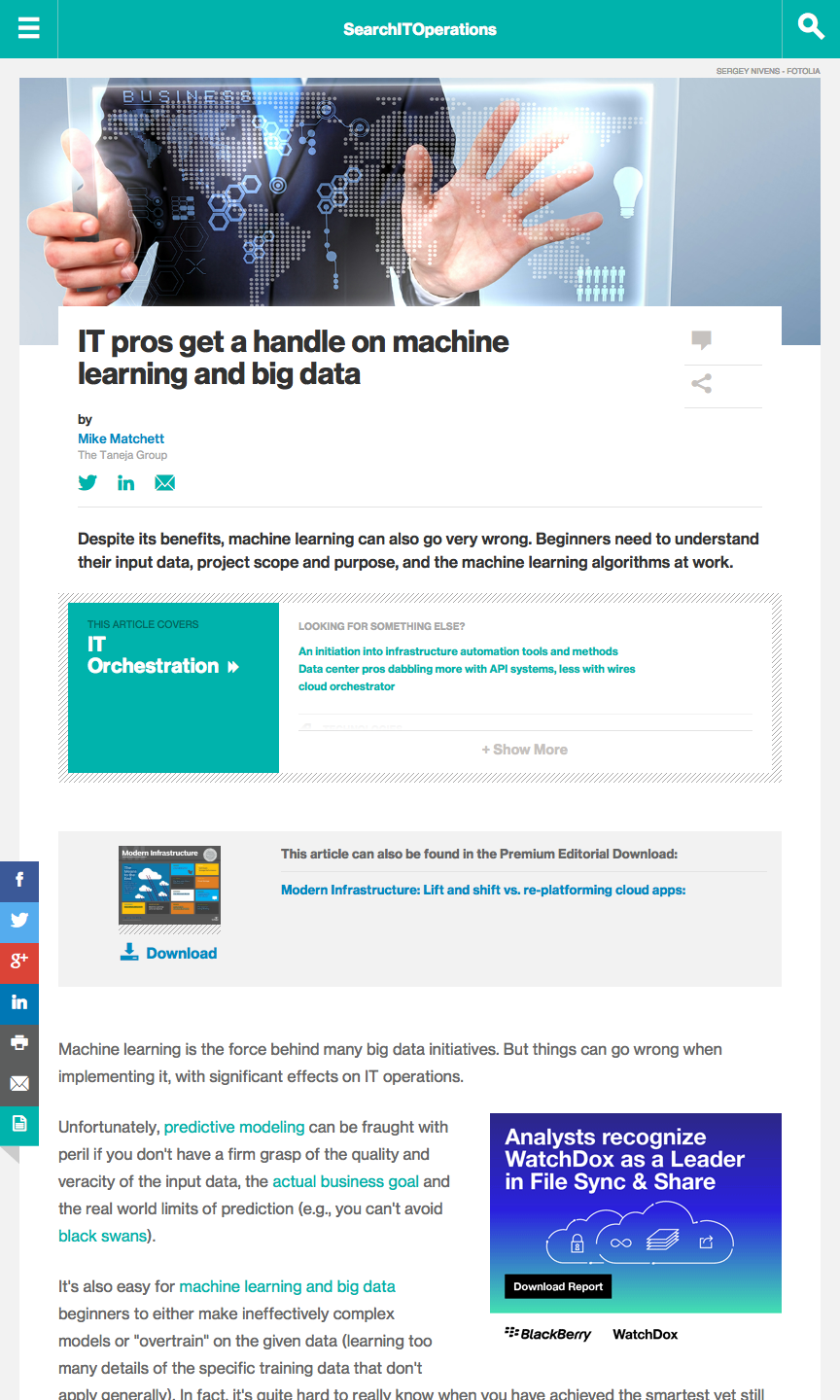
An IT industry analyst article published by SearchDataCenter.
Machine learning is the force behind many big data initiatives. But things can go wrong when implementing it, with significant effects on IT operations.
Unfortunately, predictive modeling can be fraught with peril if you don’t have a firm grasp of the quality and veracity of the input data, the actual business goal and the real world limits of prediction (e.g., you can’t avoid black swans).
It’s also easy for machine learning and big data beginners to either make ineffectively complex models or “overtrain” on the given data (learning too many details of the specific training data that don’t apply generally). In fact, it’s quite hard to really know when you have achieved the smartest yet still “generalized” model to take into production.
Another challenge is that the metrics of success vary widely depending on the use case. There are dozens of metrics used to describe the quality and accuracy of the model output on test data. Even as an IT generalist, it pays to at least get comfortable with the matrix of machine learning outcomes, expressed with quadrants for the counts of true positives, true negatives, false positives (items falsely identified as positive) and false negatives (positives that were missed).
…(read the complete as-published article there)