Data lakes swim with golden information for analytics
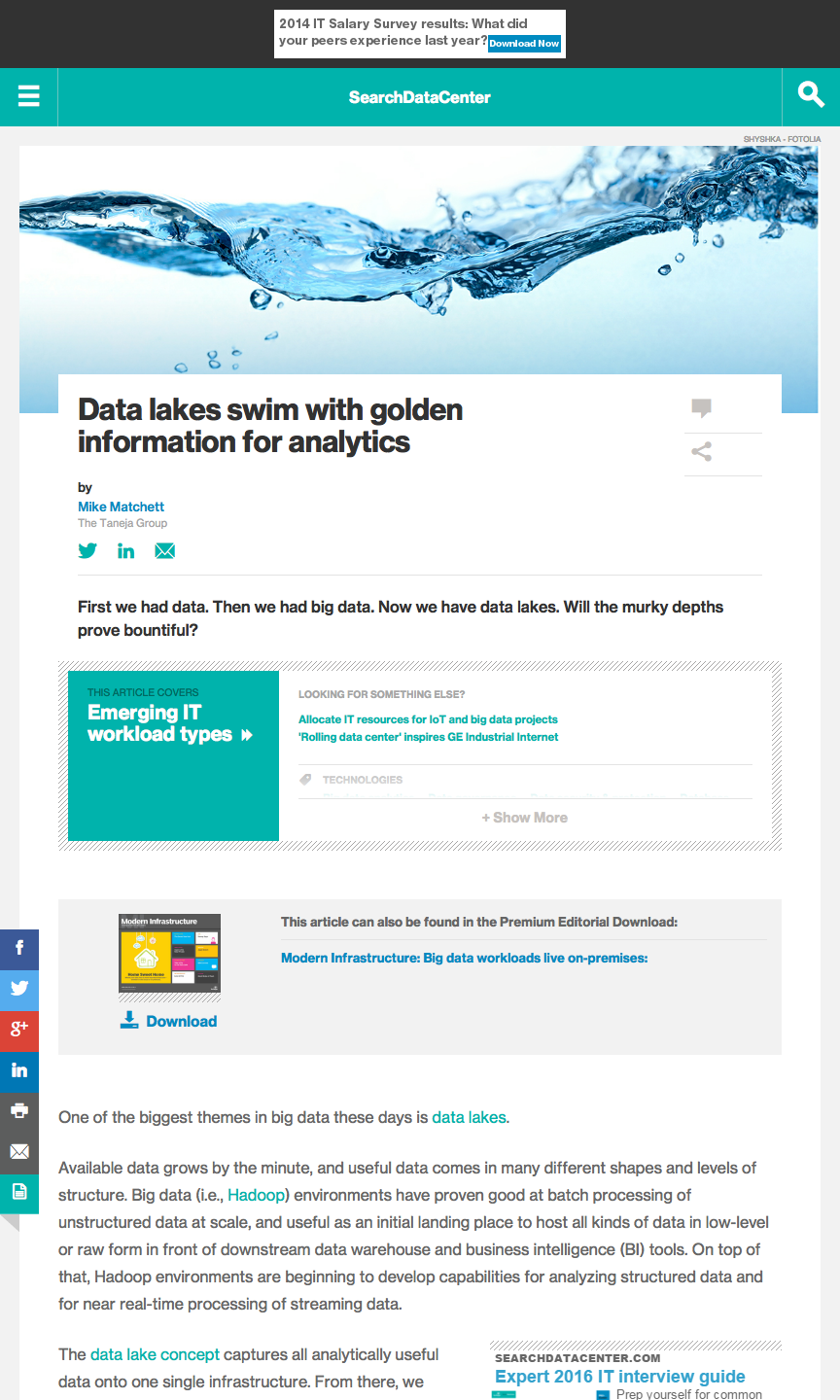
An IT industry analyst article published by SearchDataCenter.
One of the biggest themes in big data these days is data lakes.
Available data grows by the minute, and useful data comes in many different shapes and levels of structure. Big data (i.e., Hadoop) environments have proven good at batch processing of unstructured data at scale, and useful as an initial landing place to host all kinds of data in low-level or raw form in front of downstream data warehouse and business intelligence (BI) tools. On top of that, Hadoop environments are beginning to develop capabilities for analyzing structured data and for near real-time processing of streaming data.
The data lake concept captures all analytically useful data onto one single infrastructure. From there, we can apply a kind of “schema-on-read” approach using dynamic analytical applications, rather than pre-build static extract, transform and load (ETL) processes that feed only highly structured data warehouse views. With clever data lake strategies, we can combine SQL and NoSQL database approaches, and even meld online analytics processing (OLAP) and online transaction processing (OLTP) capabilities. Keeping data in a single, shared location means administrators can better provide and widely share not only the data, but an optimized infrastructure with (at least theoretically) simpler management overhead.
The smartest of new big data applications might combine different kinds of analysis over different kinds of data to produce new decision-making information based on operational intelligence. The Hadoop ecosystem isn’t content with just offering super-sized stores of unstructured data, but has evolved quickly to become an all-purpose data platform in the data center.
…(read the complete as-published article there)